Navigating with Data: Marketing Information Systems for Decision-Making
Marketing Research
Marketing research[1] refers to the systematic process of designing, collecting, analyzing, and reporting data relevant to a specific marketing situation facing an organization. It is usually conducted to address specific problems or opportunities. This process is often project-based, meaning it is initiated for a particular purpose and ends once the data has been analyzed and reported.
Roles of Marketing Research
Marketing research is used for:
- Problem-Solving: Marketing research helps organizations solve specific problems. For example, if a company is considering launching a new product, they might conduct research to understand consumer needs, preferences, and the potential demand for the product.
- Decision Support: It provides data that supports strategic and tactical decision-making. This could include decisions related to product development, pricing, distribution channels, or promotional strategies.
- Risk Reduction: By providing in-depth insights into consumer behaviour and market trends, marketing research reduces the risk associated with business decisions. For example, understanding why a previous product launch failed can help prevent similar mistakes in the future.
Marketing Research Process
The marketing research process typically involves the following steps:
- Identify the Problem or Opportunity: Define the specific problem or opportunity that requires research. This involves understanding the underlying issues or questions that need to be addressed.
- Example: A company might identify a problem such as declining sales and seek to understand the reasons behind it.
- Develop a Research Plan: Outline the research design and methodology. This includes deciding on the type of data needed, research methods (e.g., surveys and interviews), and how the data will be collected and analyzed.
- Example: A company’s research plan may involve conducting surveys to gather customer feedback or analyzing sales data to identify trends.
- Collect Data: Gather the necessary data using the chosen research methods. This can involve primary data collection (e.g., surveys and focus groups) or secondary data collection (e.g., existing reports and industry data).
- Example: A company might conduct online surveys to collect customer opinions about a new product feature.
- Analyze Data: Process and analyze the collected data to identify patterns, trends, and insights. This step involves using statistical tools and techniques to draw meaningful conclusions.
- Example: A company might analyze survey responses to determine customer satisfaction levels and identify areas for improvement.
- Present Findings: Compile the research findings into a clear and actionable report. This includes visualizing data through charts and graphs and providing recommendations based on the insights.
- Example: A company might present a report that highlights key customer preferences and suggests changes to product offerings.
- Make Informed Decisions: Use the research findings to make informed decisions and implement strategies. This involves taking action based on the insights gained to address the identified problem or opportunity.
- Example: Based on the research findings, a company might decide to revamp its product line, introduce new features that better align with customer preferences, or launch a targeted marketing campaign to regain lost customers.
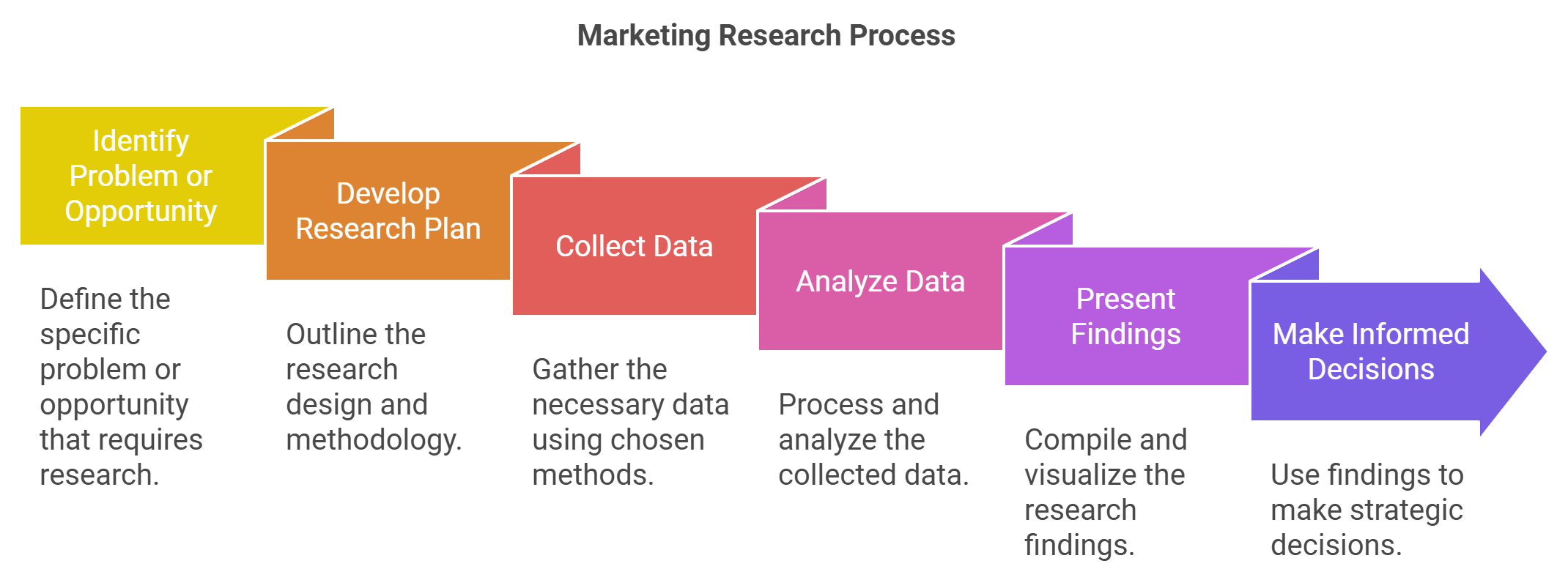
A Closer Look at Data in Marketing Research
Data is the cornerstone of marketing research, providing the insights needed to understand market dynamics, consumer behaviour, and the competitive landscape. The effectiveness of any marketing research effort largely depends on the quality, relevance, and accuracy of the data collected. To collect this data, marketers must understand where to find it, whether it is sourced internally or externally, and whether it is primary or secondary data.
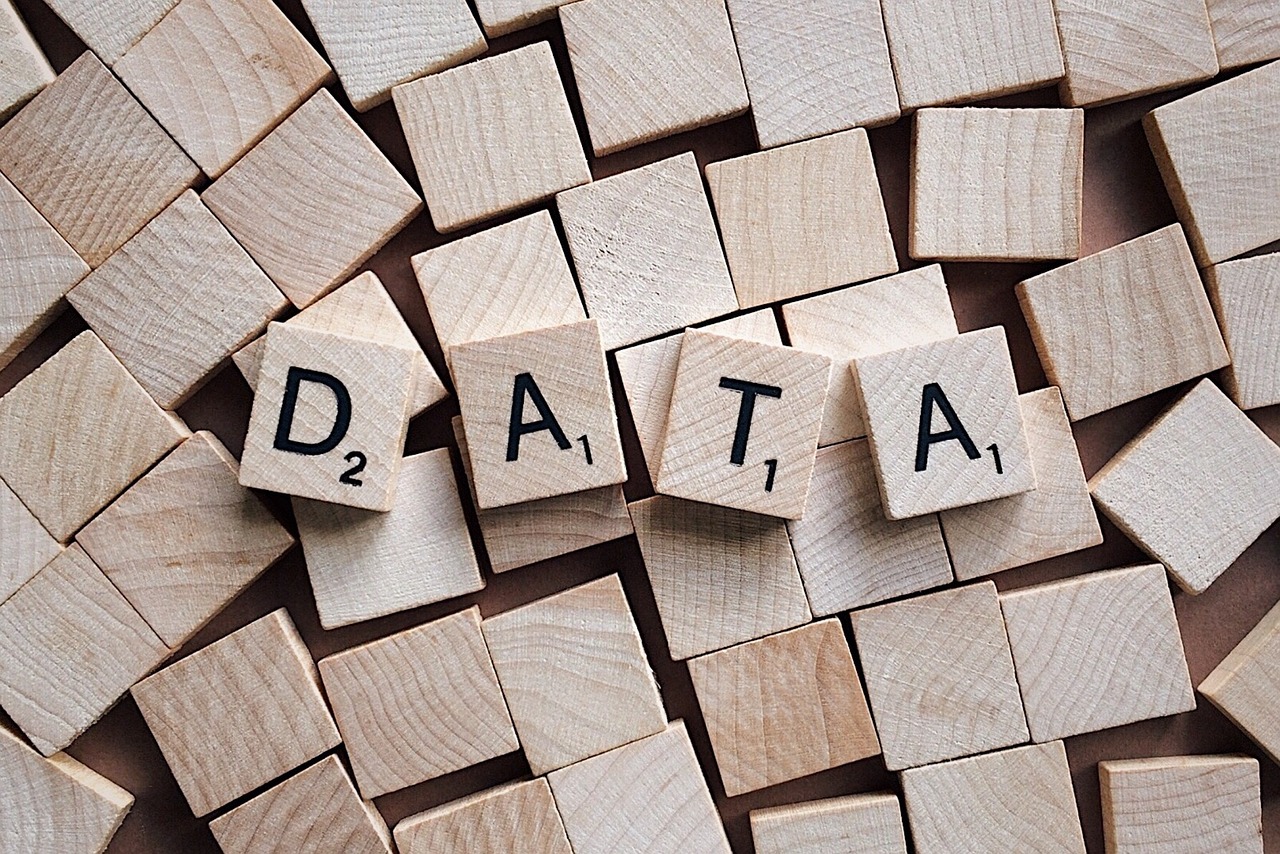
Where to Find Data?
Data can be sourced from a variety of locations, both within an organization (internally) and from outside sources (externally). The choice between internal and external data sources depends on the research objectives, the availability of data, and the specific needs of the project.
Internal Data Sources
Internal data is already available within the organization. It is often easily accessible, and it is typically collected during the normal course of business operations.
- Sales Records: Detailed data on sales transactions, including what was sold, when, where, and to whom. Sales data can provide insights into customer preferences, peak buying times, and product performance.
- Customer Databases: Information gathered from customer interactions, such as purchase history, demographic details, customer feedback, and loyalty program data. This data can help in segmenting the market and targeting specific customer groups more effectively.
- Financial Records: Financial documents like income statements, balance sheets, and cash flow statements can provide insights into the company’s performance and help identify trends or issues that may require further investigation.
- Website Analytics: Data from the company’s website, such as visitor behaviour, traffic sources, and conversion rates. Web analytics can provide insights into online consumer behaviour, the effectiveness of digital marketing campaigns, and areas for improvement.
Example
Internal Data
A retail company might use its internal sales records to identify which products are most popular during the holiday season. This internal data can then inform inventory decisions, promotional strategies, and future product launches.
External Data Sources
External data is collected from outside the organization and can provide additional context or information that is not available internally. This data is often used to complement internal data, offering a more comprehensive view of the market environment.
- Public Sources: Data from government publications, industry reports, census data, and public records. These sources are often free or low-cost and can provide valuable economic, demographic, or regulatory information.
- Commercial Sources: Data purchased from research firms, industry associations, or data vendors. This can include market reports, syndicated studies, consumer panel data, and competitor analysis.
- Social Media and Online Reviews: Information gathered from social media platforms, forums, and online reviews. This data can offer real-time insights into consumer opinions, trends, and sentiment.
- Competitor Websites and Publications: Data collected from competitors’ websites, press releases, and marketing materials. This can provide insights into competitors’ strategies, product offerings, and market positioning.
Examples
External Data
A tech company might purchase an industry report from a market research firm to understand the broader trends in the smartphone market. This external data can help the company benchmark its performance against competitors and identify emerging opportunities.
Primary vs. Secondary Data
When conducting marketing research, data can be also be categorized into primary data and secondary data. The choice between primary and secondary data depends on the research objectives, the availability of existing information, and the resources available for the research.
Primary Data
Primary data is data that is collected specifically for the research project at hand. It is original, first-hand information gathered directly from the source. Primary data collection is often necessary when existing data does not adequately address the research questions or when highly specific insights are required.
Methods of Data Collecting:
- Surveys and Questionnaires: Structured tools used to gather data from a large number of respondents. These can be conducted online, via phone, or in person.
- Interviews: In-depth, one-on-one conversations that allow for detailed exploration of individual opinions and experiences.
- Focus Groups: Group discussions moderated by a researcher to explore consumer attitudes and perceptions in a more interactive setting.
- Experiments: Controlled tests where variables are manipulated to observe the effects on outcomes, often used to test marketing strategies or product features.
- Observations: Watching and recording consumer behaviour in natural settings, such as in-store or during product usage, to gain insights into how consumers interact with products.
Advantages:
- Customized to specific research needs, providing highly relevant data
- Allows for the collection of up-to-date and accurate information
- Provides deeper insights into consumer motivations and behaviours
Disadvantages:
- Can be time-consuming and costly to collect
- Requires careful design and execution to ensure data validity and reliability
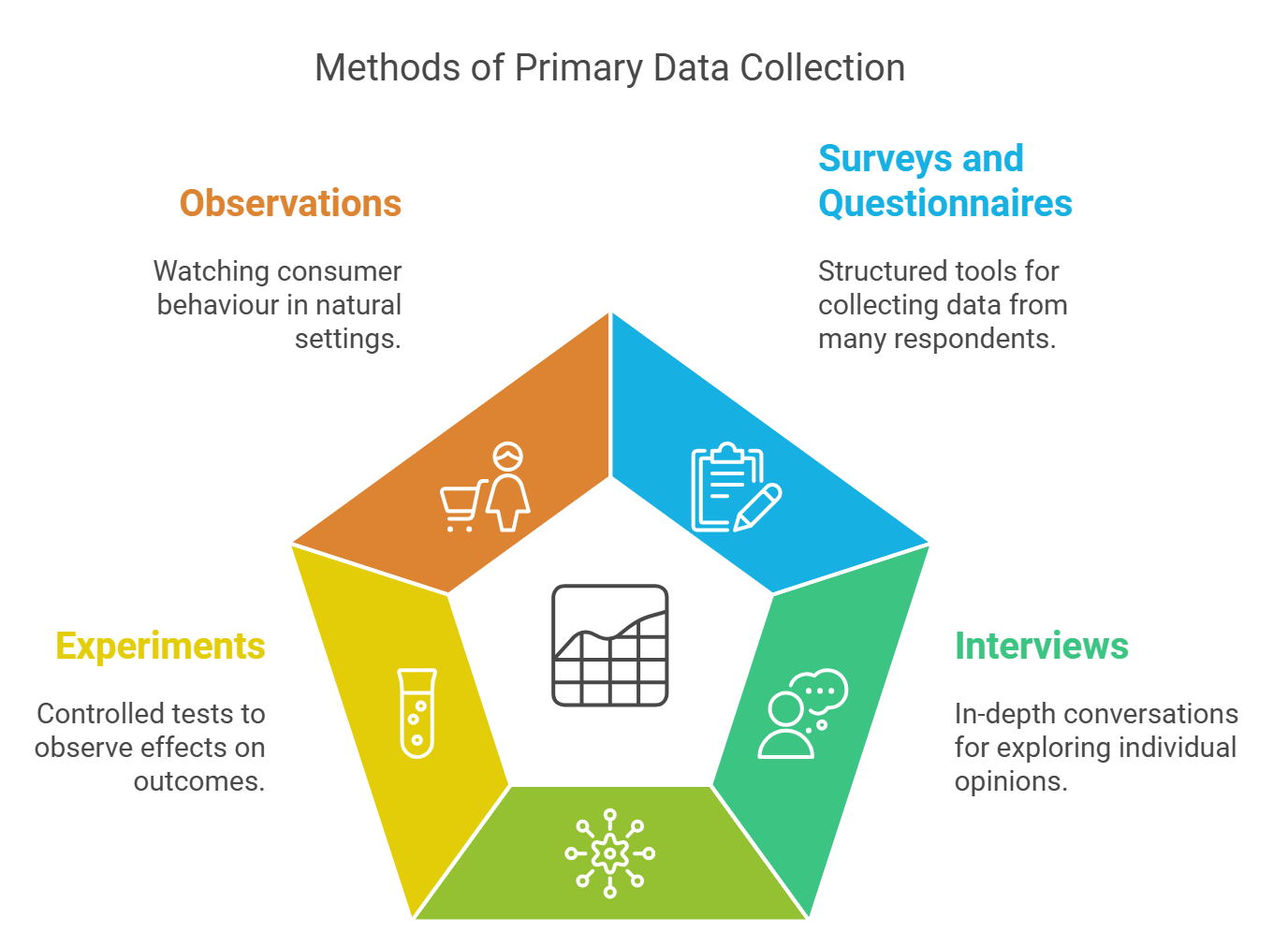
Example
Primary Data
A food company launching a new snack might conduct focus groups to gather primary data on consumer reactions to different flavors and packaging options.
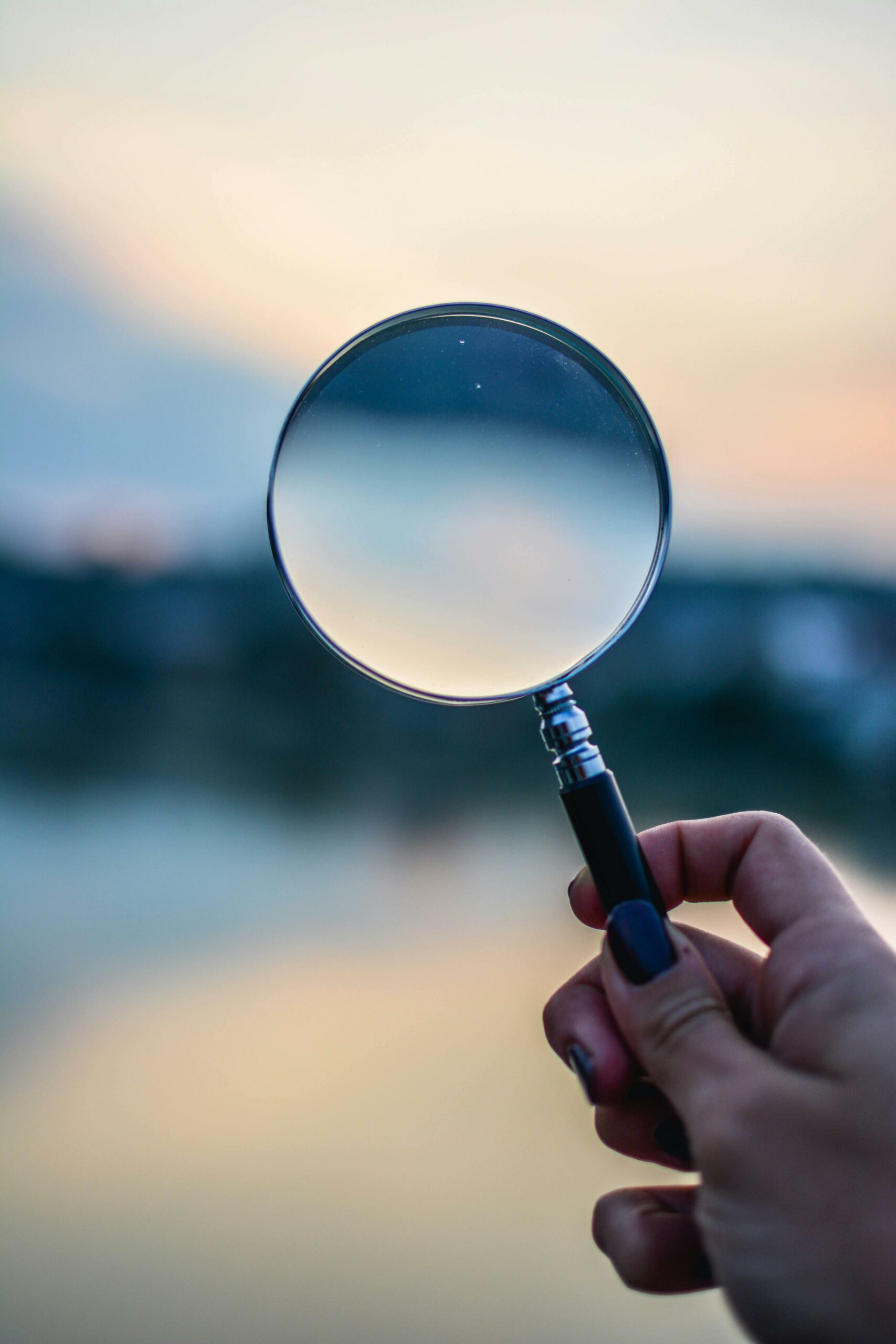
Challenges and Problems With Primary Data Collection
Primary data collection is essential for obtaining firsthand information tailored to specific research needs. However, it can present several challenges and problems that can affect the quality and reliability of the data collected.
Here are some common problems associated with primary data collection:
Bias and Sampling Issues:
- Selection Bias: This bias occurs when the sample is not representative of the entire population, leading to skewed results. For instance, if a survey is conducted only among a specific demographic group, the findings may not accurately reflect the views of the broader population.
- Response Bias: Participants may provide socially desirable answers or inaccurately recall information, which can distort the data. This is common in self-reported data where individuals might not disclose true behaviours or opinions.
Cost and Time Constraints:
- Resource-Intensive: Collecting primary data can be expensive and time-consuming, requiring significant financial investment in designing data collection tools, training personnel, and compensating participants. This can be particularly challenging for small organizations or researchers with limited budgets.
- Extended Timelines: The process of planning, collecting, and analyzing primary data often involves lengthy timelines, which can delay the availability of results and subsequent decision-making.
Data Quality and Management:
- Inaccurate or Incomplete Data: Data collected may be inaccurate or incomplete due to errors in data entry, misunderstandings during data collection, or participants not fully completing surveys or interviews.
- Data Management Challenges: Once collected, data must be organized and stored systematically to ensure integrity. Poor data management can lead to loss of data or difficulties in analysis.
Participant Recruitment and Response Rates:
- Recruitment Challenges: Finding a sufficient number of participants who meet the study criteria can be difficult, especially for niche or hard-to-reach populations. This can impact the representativeness and generalizability of the findings.
- Low Response Rates: Ensuring a high response rate can be challenging, particularly in survey-based research. Low response rates can introduce bias and limit the reliability of the data.
Ethical and Privacy Concerns:
- Informed Consent: Ensuring that individuals are fully informed about how their data will be used and have consented to its collection is a fundamental ethical requirement. This includes clear communication about the purpose, scope, and implications of data collection.
- Compliance with Privacy Laws: In Canada, privacy is protected by laws such as the Privacy Act[2] and the Personal Information Protection and Electronic Documents Act (PIPEDA)[3]. These laws regulate how personal information is collected, used, and disclosed by both public and private sector organizations.
Data Breaches and Unauthorized Access: Organizations must implement robust security measures to prevent data breaches and unauthorized access to personal information.
Secondary Data
Secondary data refers to information that was collected for another purpose but can be repurposed for the current research project. This data is usually already available and can be internal (within the organization) or external (from public or commercial sources).
Sources:
- Internal Sources: Company records, past marketing research reports, sales data, and customer databases.
- External Sources: Government reports, industry publications, academic journals, news articles, and commercial databases.
Advantages:
- Generally quicker and less expensive to obtain than primary data
- Provides a broad context or background for the research problem
- Useful for trend analysis, benchmarking, and comparative studies
Disadvantages:
- May not be perfectly aligned with the current research needs
- Can be outdated or lack relevance to the specific research questions
- Can vary in quality and accuracy depending on the source
Example
Secondary Data
A company considering entering a new geographic market might use secondary data from government census reports and industry publications to assess the market potential and demographic profile of the region.
Qualitative and Quantitative Data in Marketing Research
Data can also be broadly categorized into two types: qualitative and quantitative data. Each type has its strengths and is used in different contexts depending on the research objectives.
Qualitative Data
Qualitative data is non-numerical information that provides insights into the underlying reasons, opinions, and motivations behind consumer behaviour. It is descriptive and exploratory in nature, often focusing on the “why” and “how” of decision-making processes rather than the “what” or “how much.” This type of data is typically collected through methods that involve open-ended responses, allowing for a deeper exploration of complex issues.
Characteristics:
- Descriptive: Focuses on describing attributes, characteristics, or experiences.
- Exploratory: Used to explore underlying reasons, motivations, or perceptions.
- Subjective: Interpretation of data is often influenced by the researcher’s perspective.
- Unstructured: Data is collected in a flexible, open-ended format.
Methods of Data Collecting:
- Interviews: One-on-one discussions where participants share their thoughts and experiences in depth. For example, a researcher might interview customers to understand their experiences with a particular brand.
- Focus Groups: Group discussions led by a moderator to explore participants’ attitudes and perceptions about a product, service, or concept. Focus groups can reveal group dynamics and collective viewpoints.
- Observations: Recording behaviours and interactions in natural settings, such as observing how shoppers navigate a store or interact with a product display.
- Open-Ended Surveys: Questionnaires that allow respondents to answer in their own words, providing rich, detailed responses that reveal underlying attitudes and feelings.
Advantages:
- Depth of Understanding: Provides deep insights into consumer motivations, attitudes, and behaviours that quantitative data might overlook.
- Flexibility: Allows researchers to explore unexpected topics or themes that arise during data collection.
- Contextualization: Helps in understanding the context of consumer decisions, including cultural, social, and emotional factors.
Disadvantages:
- Subjectivity: Interpretation can be influenced by researcher bias, and different researchers may draw different conclusions from the same data.
- Time-Consuming: Data collection and analysis can be time-intensive, especially for in-depth interviews or focus groups.
- Limited Generalizability: Findings from qualitative data are often based on small, non-representative samples, making it difficult to generalize results to a broader population.
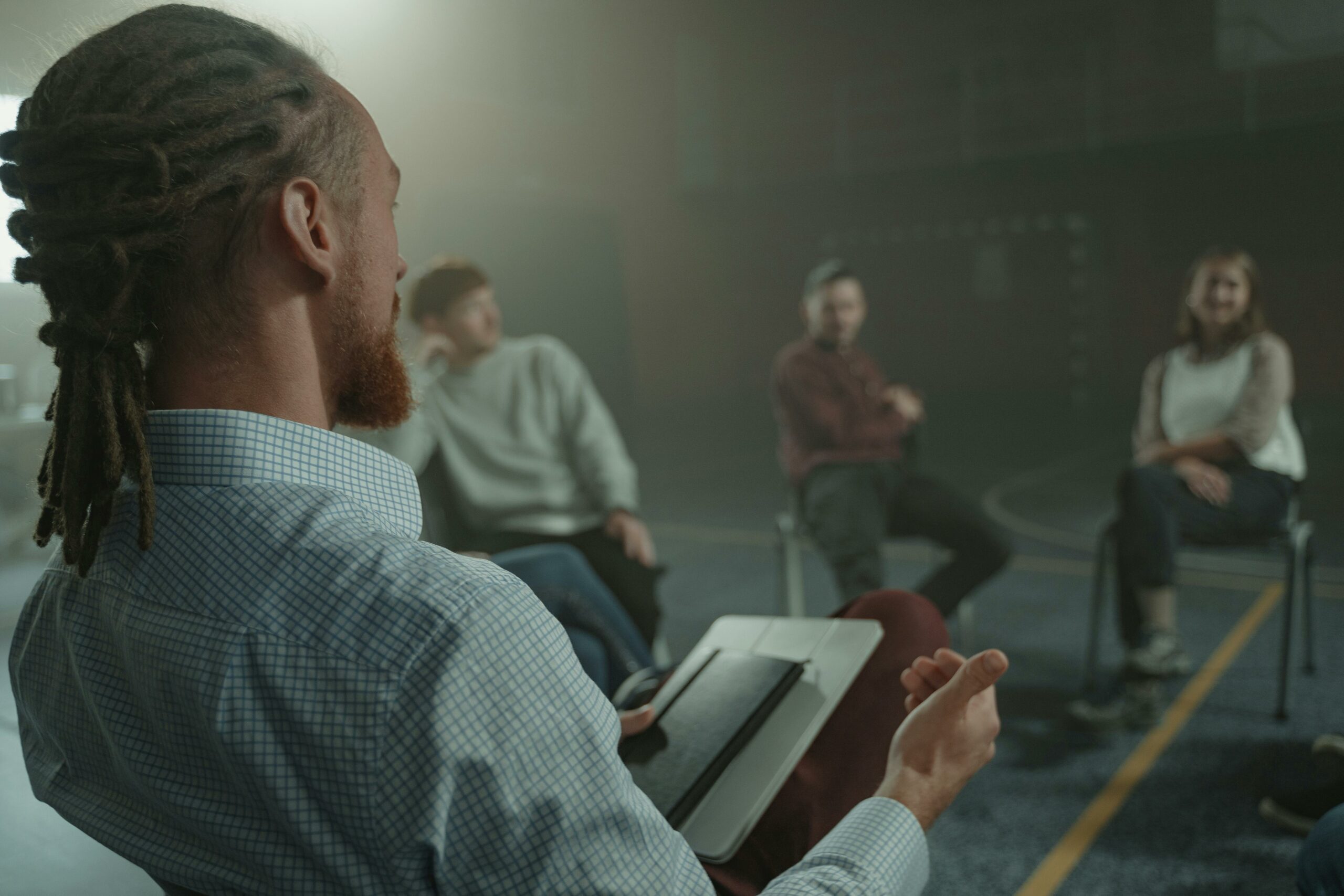
Example
Qualitative Data
A company launching a new skincare line might conduct focus groups with potential customers to gather qualitative data on their skincare routines, preferences, and feelings about different product ingredients.
Quantitative Data
Quantitative data is numerical information that can be measured and analyzed statistically. It focuses on quantifying behaviours, opinions, and attitudes — providing data that can be used to identify patterns, trends, and correlations. This type of data is typically collected through structured methods that allow for precise measurement and comparison.
Characteristics:
- Numerical: Data is expressed in numbers, such as counts, percentages, or averages.
- Objective: Results are less subject to researcher bias because they rely on standardized measurements.
- Structured: Data collection follows a predetermined format, often with closed-ended questions.
- Statistical: Analysis involves statistical methods to test hypotheses, identify trends, or make predictions.
Methods of Data Collecting:
- Surveys: Structured questionnaires with closed-ended questions (e.g., multiple-choice, rating scales) that can be distributed online, by phone, or in person. Surveys are widely used to gather data from large populations.
- Experiments: Controlled studies where variables are manipulated to observe their effect on outcomes. For example, a company might test different price points to determine the optimal pricing strategy.
- Observational Research: Counting and recording specific behaviours, such as the number of customers entering a store or the time spent on a website.
- Analytics: Analyzing data from digital platforms — such as website traffic, social media engagement, or sales figures — to identify trends and patterns.
Advantages:
- Precision: Provides exact measurements that can be statistically analyzed.
- Generalizability: Large, representative samples allow findings to be generalized to a broader population.
- Objectivity: Standardized data collection methods reduce the influence of researcher bias.
Disadvantages:
- Limited Depth: Quantitative data may lack the context or depth needed to fully understand complex behaviours or motivations.
- Inflexibility: Structured data collection methods may miss important insights that fall outside the scope of predefined questions.
- Requires Large Samples: To ensure statistical significance, quantitative research often requires large sample sizes, which can be costly and time-consuming to obtain.
Example
Quantitative Data
A fast-food chain might use quantitative data collected from customer satisfaction surveys, where respondents rate their experience on a scale from 1 to 10. The data could then be analyzed to determine the average satisfaction score and identify areas for improvement.
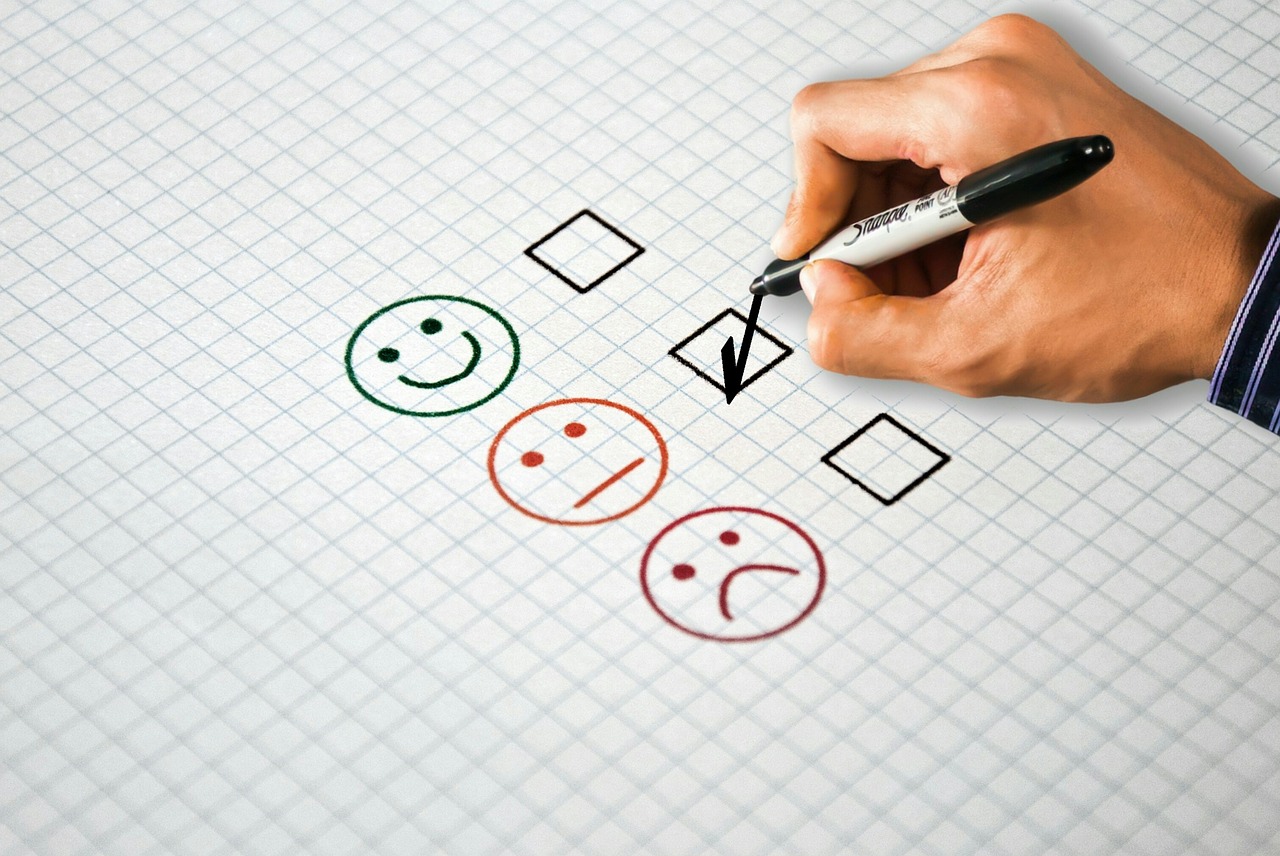
Both qualitative and quantitative data are important in marketing research. Qualitative data offers contextual insights, helping to understand the “why” behind decisions. In contrast, quantitative data provides measurable, numerical insights that allow marketers to quantify trends and make data-driven decisions. By combining both types of data, marketers can gain a well-rounded understanding of the relevant issues, leading to more effective and informed decisions.
Emerging Marketing Research Methods and Practices
Marketing research methods and practices are evolving rapidly[4], driven by technological advancements. Here are some notable emerging trends and practices[5].
Artificial Intelligence and Automation
Artificial intelligence (AI) will continue to revolutionize marketing research by enabling hyper-personalization, predictive analytics, and automation of repetitive tasks. AI tools such as chatbots, advanced data analytics, and machine learning algorithms will help businesses predict consumer behaviour, optimize campaigns, and personalize customer experiences at scale. For example, AI-driven tools can analyze customer data to recommend tailored product offerings or predict market trends with improved accuracy.
Example
AI and Automation
Scenario: Imagine you are part of a marketing team at an e-commerce company like Amazon. Your team uses an AI-powered tool to analyze customer purchase history and browsing behaviour. The AI identifies that customers who buy fitness trackers are also likely to purchase protein supplements.
Based on this insight, your team creates a targeted email campaign offering discounts on protein products to fitness tracker buyers, resulting in a 20% increase in sales.
Digital Qualitative Research
The shift from traditional qualitative methods (e.g., focus groups) to digital platforms (i.e., digital qualitative research) is accelerating. Online tools and social media analytics allow researchers to gather deep insights into consumer behaviour more efficiently and cost-effectively. This trend supports inclusivity by enabling diverse participation while reducing biases associated with physical settings.
Example
Digital Qualitative Research for Travel Trends
Scenario: A travel agency conducts virtual focus groups with participants from different countries to understand post-pandemic travel preferences. Using video conferencing tools, they discover that travelers are prioritizing eco-friendly destinations and wellness retreats.
Based on these insights, the agency curates travel packages that emphasize sustainability and relaxation.
Synthetic Data for Privacy and Efficiency
Synthetic data is emerging as a solution to challenges like privacy concerns, data scarcity, and survey fatigue. This artificial data mimics real-world responses, allowing researchers to conduct faster and more cost-effective studies while protecting sensitive information.
Example
Synthetic Data for Destination Marketing
Scenario: A tourism board wants to promote a new destination but lacks sufficient visitor data due to its recent launch. By using synthetic data, the board simulates traveler profiles and preferences, enabling them to design targeted advertising campaigns. For instance, they identify that young professionals might be interested in adventure activities, while families prefer cultural experiences, and tailor their marketing accordingly.
Media Attributions
- Figure 1: “The marketing research process” [created using Napkin.ai] by the author is under a CC BY-NC-SA 4.0 license.
- Figure 2: “Data, Letters, Scrabble” by WOKANDAPIX (2017), via Pixabay, is used under the Pixabay content license.
- Figure 3: “Methods of primary data collection” [created using Napkin.ai] by the author is under a CC BY-NC-SA 4.0 license.
- Figure 4: “Selective Focus Photo of Magnifying Glass” by lil artsy (2018), via Pexels, is used under the Pexels license.
- Figure 5: “A Man Sitting on a Chair Holding a Notebook in Front of a Group of People” by Tima Miroshnichenko (2020), via Pexels, is used under the Pexels license.
- Figure 6: “Feedback, Survey, Nps” by Mohamed Hassan [Mohamed_hassan] (2018), via Pixabay, is used under the Pixabay content license.
- Malhotra, N. K. (2020). Marketing research: An applied orientation (7th ed.). Pearson. ↵
- Office of the Privacy Commissioner of Canada. (2019, September 6). The privacy act. https://www.priv.gc.ca/en/privacy-topics/privacy-laws-in-canada/the-privacy-act/ ↵
- Office of the Privacy Commissioner of Canada. (2021, December 8). Personal information protection and electronic documents act (PIPEDA). https://www.priv.gc.ca/en/privacy-topics/privacy-laws-in-canada/the-personal-information-protection-and-electronic-documents-act-pipeda/ ↵
- Thakur, J., & Kushwaha, B. P. (2024). Artificial intelligence in marketing research and future research directions: Science mapping and research clustering using bibliometric analysis. Global Business and Organizational Excellence, 43(3), 139–155. https://doi.org/10.1002/joe.22233 ↵
- Henriques, A. (2024, October 22). The 4 market research trends redefining insights in 2025. Qualtrics. https://www.qualtrics.com/blog/market-research-trends/ ↵
Tools and methods used to measure and analyze website traffic and user behaviour.
Original data collected specifically for a research project through methods like surveys, interviews, or experiments.
A research method using structured questions to collect data from a sample of respondents.
A qualitative research method involving one-on-one conversations to gather detailed information about opinions, experiences, and preferences.
A qualitative research method where a moderator leads a small group discussion to gather insights about products, services, or concepts.
A research method where variables are controlled and manipulated to test cause-and-effect relationships in marketing scenarios.
A method of collecting data by watching and recording behaviour in natural settings without direct interaction.
A systematic error in data collection that can distort research findings, such as selection bias (non-representative sampling) or response bias (inaccurate participant responses).
Pre-existing data collected for another purpose but repurposed for current research needs. Examples include government reports or industry publications.
Non-numerical information that explores motivations and attitudes through methods like interviews or focus groups.
Numerical information that can be measured and analyzed statistically to identify patterns and trends.
The use of computer systems to simulate human intelligence, enabling tasks such as data analysis, predictive modeling, and automation in marketing research.
The use of online tools and platforms to collect qualitative data, such as virtual focus groups or social media analytics.
Artificially generated data that mimics real-world responses while addressing privacy concerns and data scarcity.